The Center for Security and Emerging Technology (CSET) at Georgetown University’s Walsh School of Foreign Service released a May 2020 issue brief examining artificial intelligence (AI) and machine learning (ML) from a cybersecurity perspective with a list of questions for policymakers to consider.
The brief, A National Security Research Agenda for Cybersecurity and Artificial Intelligence by Director of the Cybersecurity and AI Project Ben Buchanan, walks policymakers through the “machine learning paradigm of artificial intelligence” with a focus on the unknowns of ML offense, defense, adversarial learning, and more.
Buchanan recommends a thorough study of the ML “kill chain,” or steps that hackers will use to achieve their goals, to help network defenders and software engineers find and remediate possible vulnerabilities.
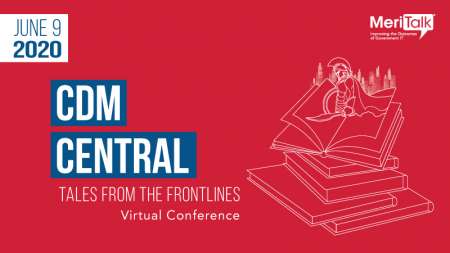
“One of the foremost national security questions at the intersection of cybersecurity and AI is the degree to which machine learning will reshape or supercharge this kill chain,” he wrote. “There are reasons for concern, but also reasons to think present-day automation—not using machine learning techniques—is already effective in human-machine teams.”
He also asks policymakers to reflect on other offensive issues, such as how ML could be used to tailor and scale spear phishing attempts and make cyber capabilities more powerful. Buchanan goes on to address the defense use of ML, specifically how it could be used to detect malicious code or more effectively attribute cyberattacks.
“If machine learning can improve detection, interdiction, and attribution, it can dramatically reduce the potential dangers of cyber operations,” the brief states, but clarifies that evaluation should be grounded in practical and measurable results.
Buchanan also addresses the concern that just like traditional computer systems, ML comes with its own weaknesses such as software bugs and fundamental vulnerabilities that provide hackers with new opportunities to exploit the system. He wrote that policymakers should be prepared to consider how ML can be secured against these attempts at deception or unintentionally reveal secrets if trained with classified data.
While most of the brief focuses on the technical questions associated with ML application, Buchanan concludes by asking policymakers to keep in mind other overarching questions about the relationship between AI and national security.
“Moreover, at least in the near term, machine learning capabilities will add complexity to traditional attack vectors, raising the risks that cyber operators may adopt machine learning features without fully understanding their inner workings or potential effects,” he cautions.
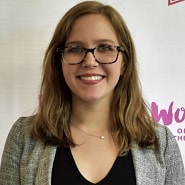