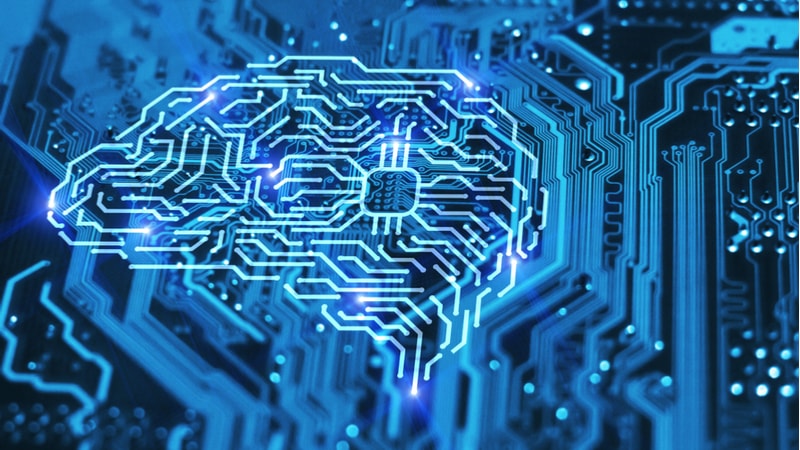
A panel of Federal agency officials who are knee-deep in implementing machine learning (ML) and artificial intelligence (AI) technologies explained critical steps to getting started with the technologies, and dealing with data-bias issues, at an event organized by ATARC on Oct. 24.
The panelists highlighted some early wins in deployments of AI/ML tech, but also emphasized the importance of investing in proper data preparation to pave the way for good results.
Prep Work Called Essential
Bennett Gebken, Senior Program Analyst in the Veterans Affairs Department’s (VA) Office of Business Process Integration, said the VA is starting to undertake some of the substantial data-prep work necessary to do more with machine learning (ML) technologies. “We have a lot of unstructured data and we are starting to clean that up to make better classifications,” he said.
Edward Dowgiallo, Principal Solutions Architect at the Department of Transportation (DOT), said a large part of successful AI/ML strategy execution involves the more mundane tasks of cleaning and structuring data so that it is usable for those technologies. “About 95 percent of the work is cleaning and structuring, and the other five percent is analysis,” he said. He also recommended that agencies create unified operations for using AI/ML, so that different segments of agencies don’t have to repeat a lot of the preliminary work.
Early Forays
Reza Rashidi, Lead IT Executive for Robotics Process Automation at the Internal Revenue Service (IRS), said his agency is already using AI technologies for compliance-related tasks, and is exploring further use for customer service and experience applications. Chat-box customer service applications, for example, can yield the important benefit of freeing up customer service reps for more complicated assistance requests, he said.
Dowgiallo said DoT has been working with ML technologies for more than a year with the aim of replacing rules-based systems, and has been successful in doing away with 200 of the 1,400 rules involved in the project. Based on that result, “we are looking at moving that [technology] into the rest of the system,” he said.
He recommended that AI/ML implementers “start in one area, learn it really well,” and then splice those results and experience into related areas.
Todd Myers, Automation Lead at the National Geospatial-Intelligence Agency (NGA), said his agency has been using ML technologies for about four years and is reaping the benefits of that work, including using ML to quickly perform analysis that used to take months. He also said NGA is keeping its eye on the progress of quantum computing technologies as the “quantum cubit will change” AI/ML technologies.
Gil Alterovitz, Director of Artificial Intelligence at VA, recommended that agencies first “think about your use case” for AI technology, then find an AI product that fits that bill. If an agency can’t find an existing product, “you are going to have to invent that, which can cost a lot of money,” he said.
Gebken agreed, saying that the very first step in the process should be understanding compelling business purposes for deploying the technology. “We should not do AI just for the sake of doing AI,” he advised.
“You don’t go out and buy AI and algorithms,” said Myers, who continued, “You build it and curate it against your data sets.” The proof of the technology’s value, he said, is whether it improves an organization’s data analytics.
Dealing with Data Bias
Concerns about data bias in ML/AI algorithms – which can produce skewed outputs and lead to subsequent flawed analysis and decision-making – were also top of mind for the Federal panelists.
Algorithms, said Dowgiallo, “are going to miss on certain points, and will need to be redefined.” In those cases, he said, it’s time to “break apart” the algorithm in order to find its flaws. Countering data bias, he said, “is about understanding the math and understanding what you are doing with it . . . You’re not going to get it perfect all the time.”
“The takeaway is we still have to have a human to validate” the detection of bias flags, said Myers. Bennett agreed, saying that “the human in the loop” remains a firm requirement.