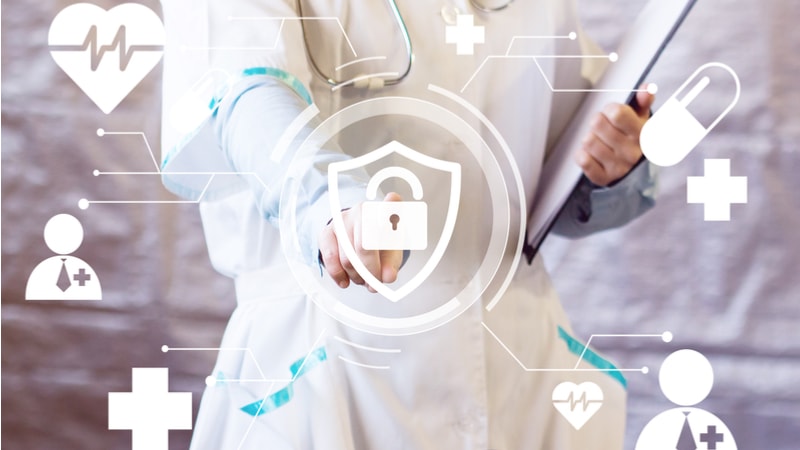
The use of artificial intelligence to improve health outcomes offers great promise, but members of Congress and Capitol Hill witnesses warned this week that the emerging technology can introduce bias without the use of diverse data and reinforcement learning staff that helps in training AI models.
AI is only as good as its input information, as the technology relies heavily on the quality of training data that teaches the AI models to make decisions, according to experts who testified before a Senate Health Education, Labor, and Pensions subcommittee on Wednesday.
“It seems to me that AI has a diversity problem,” said Sen. Ben Ray Luján, D-N.M. “The way I’m looking at this is we need technology to help improve health outcomes, reduce health disparities – not exacerbate them – and it’s clear that AI has the power to do both. Which points me to the realization that AI is only as good as its inputs.”
For example, Sen. Luján pointed to studies finding that AI trained on chest X-rays mostly from male patients will perform poorly when a doctor applies it to a female patient. Additionally, he said an algorithm designed to diagnose skin cancer will botch the diagnosis if the patient is dark skinned and most of the training images come from fair skinned patients.
Dr. Kenneth Mandl, a professor at Harvard Medical School and the director of the Boston Children’s Hospital Computational Health Informatics Program, agreed that there is a diversity of data problem.
He stressed the importance of diverse data and data interoperability across health systems, so that it’s not just “the highest performing health systems that are wealthy enough to have teams in their IT departments that can extract data and make it available.”
However, Dr. Mandl also pointed to a lesser known step in the algorithm development process that could limit diversity in the large language models.
“The models are further trained after they’ve been developed on the data – which already may lack diversity. They’re trained with something called ‘reinforcement learning with human feedback,’ where people tell the AI whether it was right or wrong when answering certain questions,” Dr. Mandl explained.
“And so, we actually need a diversity of staff who are doing the reinforcement learning as well, so that we get the right mix across multiple perspectives,” he said. “It’s demonstrated over and again that lack of diversity in the data leads to biased conclusions that do not serve the full population well.”
Dr. Mandl also stressed that it’s better to train these models with diverse data and staff in the early stages, so that the models are developed with less bias at the beginning. “That bias can become entrenched and harder to fix later,” he noted.
Similarly, Dr. Thomas Inglesby, the director of the Johns Hopkins Center for Health Security, told senators that in order to realize the health benefits of AI, the risks must be addressed.
“AI offers great potential benefits for health care and public health,” Dr. Inglesby said. “However, to realize these benefits it’s vital to address potentially very serious risks. AI developers could inadvertently introduce biases into healthcare-related models.”
In order to address such risks, he said that entities developing models with significant dual-use risks should be required to red-team and evaluate their models. Additionally, he recommended .that Congress task an agency with auditing those models and submitting a report to Congress “with recommendations for new authorities that will be needed by the agency to take any appropriate remedial actions.”
“Congress should pursue these measures in a manner that will allow AI developers and scientists to continue to vigorously pursue the many very positive uses of AI to improve human health,” he concluded.