The Department of Defense’s (DoD) Defense Advanced Research Projects Agency (DARPA) announced the launch of its Guaranteeing AI Robustness Against Deception (GARD) program, which is designed to develop new defenses against adversarial attacks on machine learning (ML) models.
The program aims to respond to adversarial AI by developing a testbed to characterize different ML defenses and assess their applicability. Researchers on the program have created resources and virtual tools for the community to be able to test and verify the effectiveness of existing and emerging ML defense models.
“Other technical communities – like cryptography – have embraced transparency and found that if you are open to letting people take a run at things, the technology will improve,” GARD program manager Bruce Draper said in the announcement. “With GARD, we are taking a page from cryptography and are striving to create a community to facilitate the open exchange of ideas, tools, and technologies that can help researchers test and evaluate their ML defenses. Our goal is to raise the bar on existing evaluation efforts, bringing more sophistication and maturation to the field.”
In addition to a virtual testbed, researchers also created a toolbox, training materials, and a benchmarking dataset for the program, all included in an openly available public repository. The GARD virtual testbed, called Armory, will allow for researchers to perform “repeatable, scalable, and robust evaluations of adversarial defenses.”
The DARPA GARD team includes researchers from the University of Chicago, Google Research, IBM, MITRE, and Two Six Technologies, according to the DARPA release. Despite adversarial AI being a nascent field, researchers said they have already noticed common themes across current adversarial AI defenses. Part of the repository includes a Self-Study repository developed by Google Research, complete with “test dummies” to help evaluate defenses.
“The goal is to help the GARD community improve their system evaluation skills by understanding how their ideas really work, and how to avoid common mistakes that detract from their defense’s robustness,” Draper said. “With the Self-Study repository, researchers are provided hands-on understanding. This project is designed to give them in the field experience to help improve their evaluation skills.”
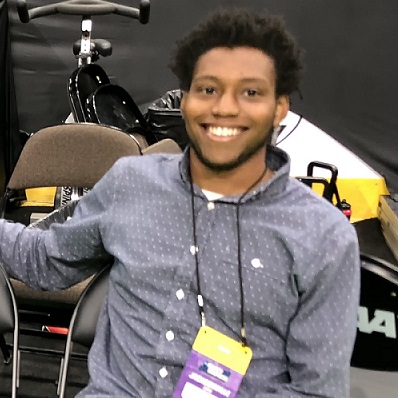