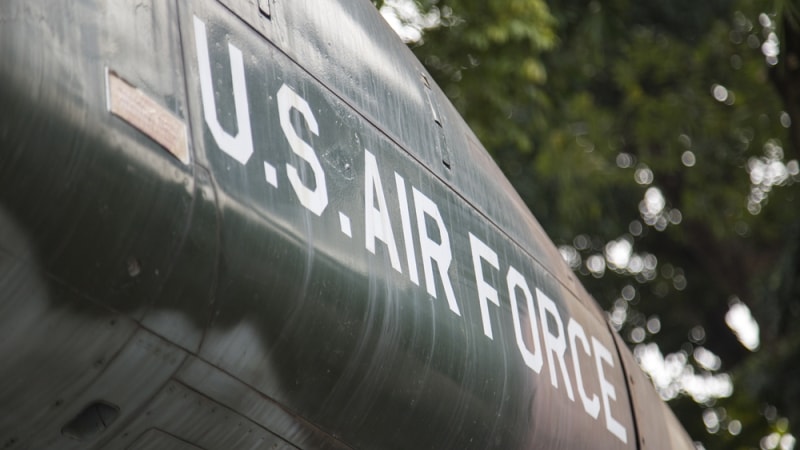
Like other military services and Department of Defense components, the Air Force is finding itself overloaded these days with streaming intelligence data, and is looking to machine learning and artificial intelligence to help its analysts quickly put all that information to practical use.
Specifically, the service is looking to fuse Multi-intelligence, or Multi-INT, which can consist of data in multiple formats from manned and unmanned aircraft, satellites, and ground stations, as well as other sources. The volume and variety of that data can leave analysts unable to parse it all and knowledgeably help inform the decision-making process. So the Air Force Research Laboratory (AFRL) has issued a Request for Information looking for input from industry, academia, and other government labs on applicable tools that are available or in development.
An overabundance of data is nothing new–the Air Force has been complaining about the dangers of sensor-driven overload since the early 2000s–but the need to solve the problem is becoming more urgent. The Air Force is moving to a new exploitation paradigm called Sense, Identify, Attribute, Share (SIAS) that requires new approaches to exploiting Multi-INT, according to the RFI.
The Air Force’s Next Generation ISR Dominance Flight Plan, signed in July this year, states that the service “must have the architecture and infrastructure to enable machine intelligence, including automation, human-machine teaming, and ultimately, artificial intelligence,” which will define the service’s Intelligence, Surveillance, and Reconnaissance (ISR) efforts going forward.
“Technology components designed to support SIAS will need to ingest, reason over, and inform both analysts and other emerging technologies designed to automate both ISR database queries and physical collection,” the RFI states.
The Air Force is far from alone in looking to use AI and machine learning to deal with the onslaught of intelligence data. The National Geospatial-Intelligence Agency (NGA) wants to use the technologies to get a handle on the massive amounts of geospatial intelligence (GEOINT) it collects, focusing on the geospatial content within its Multi-INT data sources. NGA most recently awarded seven one-year research contracts for applying advanced algorithms and machine learning to characterize geospatial data. The awards were part of the agency’s three-year Boosting Innovative GEOINT Broad Agency Announcement (BIG BAA) initiative, which since 2016 has awarded a series of contracts targeting specific topic areas.
The Department of Defense’s Project Maven is taking an algorithmic approach to analyzing millions of hours of full-motion video from drones and other sources (and was the center of controversy when some Google employees objected to the company’s involvement; Google eventually decided to leave the project). The Intelligence Advanced Research Projects Activity (IARPA) also is developing AI systems in other areas of what it calls anticipatory intelligence, such as its Deep Intermodal Video Activity (DIVA) program to automate the monitoring and analysis of endless hours of surveillance video.
But rather than focusing just on video or other individual facets of data analysis, SIAS wants to take on the whole gumbo of Multi-INT. That can involve not just fusing data from the different modes of input–such as video, imagery, communications, and other facets of signals intelligence (SIGINT) and measurement and signature intelligence (MASINT), which classifies targets–but, depending on the situation, data from open sources, like information available online, that can put signals data into context. The potential is to get at not just what and where, but why, ultimately to give the Air Force a full operating picture and the most complete situational awareness possible.
AFRL is looking for input from other DoD labs, federally funded research and development centers, academia, and industry that are working on Multi-INT and fusion capabilities in areas including the timely machine-assisted fusion of “data across multiple sources to create patterns of life and detect anomalous activity such as new tactics or procedures,” according to the RFI. Other areas include workflow management to assist decision-making, machine-assisted analysis of intelligence from a range of sources, seamless methods for providing situational awareness, and ways to dynamically share information.
To help generate interest, it will host a series of topic-specific TechFests at which potential participants can demonstrate their technologies.