Artificial Intelligence Operations (AIOps) provides a wide range of benefits for agencies, but Fed IT experts say agencies face several challenges to make the most of their AI capabilities, such as data readiness and misinformation surrounding the new technology.
During ATARC’s “Artificial Intelligence Operations-Enabling Government Agencies To Do More With Less” panel discussion on April 29, Federal IT experts discussed the challenges they face when it comes to implementing AIOps.
“Properly implementing AIOps is difficult when we in government have a constipated process by which we set up agreements with non-government entities,” said Nikunj C. Oza, leader of the data sciences group at NASA’s Ames Research Center. “We have so much of what’s been talked about with data where it’s not really ready for AI to use.”
“You can start off with trying to implement an AI project, but it screeches to a halt because the other parts of your whole operation and system are not ready for it,” he added. “So, I’m really hoping that we spend some time focusing on how we can get the other aspects of our organizations and systems set up to make best use of AI.”
Data readiness is also a barrier for AI implementation at the Department of Defense’s (DoD) Joint Artificial Intelligence Center (JAIC), according to Yevgeniya (Jane) Pinelis, chief of test and evaluation of artificial intelligence/machine learning at the JAIC.
“Data readiness is by far the biggest barrier,” Pinelis said. “I can’t tell you how many great projects get proposed at the JAIC and we just don’t have the data. Whether it be data to train, data to test, there are always some kind of issues … with label quality and data quality and data representativeness, etc.”
Another challenge when it comes to AI and machine learning is the “misinformation around AI,” Oza added.
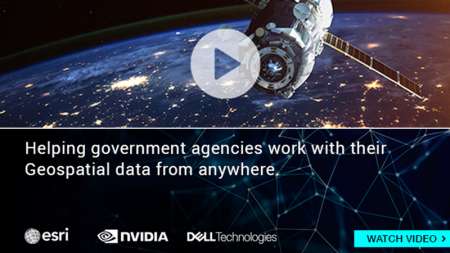
“I have unfortunately had people say that they didn’t come to our group to work on machine learning [ML] because they thought we needed a large amount of data in order to use ML. They still see ML as only deep learning, which is very data-inefficient, but there are many much more efficient methods,” Oza said. “And then you get the occasional person who will think that AI and the people like us who are implementing it are trying to go and steal their jobs or such and don’t realize that we really do view it as a partnership.”
Nevertheless, the panelists said they remain hopeful that AI will become more trustworthy over time so that they can accelerate and advance their missions.
“I hope that a year from now we’ll be that much closer to producing trustworthy AI systems,” Pinelis said. “Probably not all the way there, but hopefully considerably closer than we are today.”
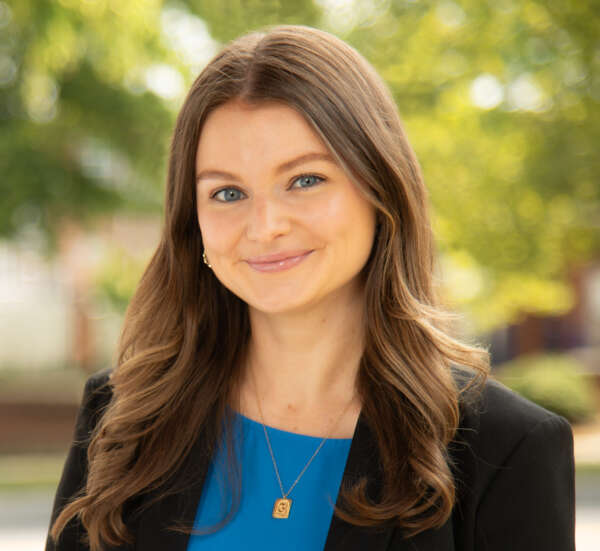