Data can’t be perfect, but it can be managed using a “food label” approach that reflects who and what the data was created for – all of which can increase the useful of data for artificial intelligence (AI) applications, a National Institutes of Health (NIH) official said.
Speaking at a NextGov/FCW event on Sept. 12, Deborah Duran, senior advisor for data science analytics and systems at NIH, said that transparency is key in data management – without it, AI systems and officials can’t understand or account for bias skews.
“The problem with this is that people think that data is just data, and that anybody can use it for anything, and that’s not true,” said Duran. “You have to have an intention and an understanding of what the data is before you develop a model, because the minute you put it in that model, and you didn’t prep the data right or have the right data set into your model, you have just now created a biased model.”
The NIH is accounting for this by creating what Duran coined a “food label” system of data management, which tells data users where the data came from and what its intended use was.
“All of you buy food, and I’m sure all of you have looked at that little food thing at the back and saw how many calories it was and what percentage of fats it was,” said Duran. “We’ve developed that same sort of look for every data set that we clean up and post, but it has on it all of the important transparency issues. What was the original source? What was done to clean it up? Who does it apply to, or what it applies to?”
“Using sort of that food label approach, it’s easy, quick – I can look at that and go, hmm, that doesn’t fit what I need, and I can move on, and if everyone does that kind of thing with their data and their models then we have a better utility to both data and models,” she continued.
Mike Horton, chief artificial intelligence officer at the Department of Transportation, said at the same event that agencies are currently “wrestling” with understanding bias skews in AI data use, noting that in addition to new data management labeling systems, the solution will require building transparency into data infrastructure.
Part of that process requires keeping a human in the loop and treating AI as “a very fast employee that has a lot of memory but is kind of dumb,” requiring that it use specific parameters and training to process the correct data.
“You have to get [the AI] smart, you have to give them the right data, you have to give them those parameters,” said Horton. “You need to make sure that you’re feeding the right stuff to it, and that it has the right veracity for what it is you’re trying to do.”
Duran warned that with predictive AI models, the imputation of data – the addition of synthetic data to already existing data sets – can cause the model to “eat itself” and requires understanding its limitations to avoid “perpetuating a bigger problem.”
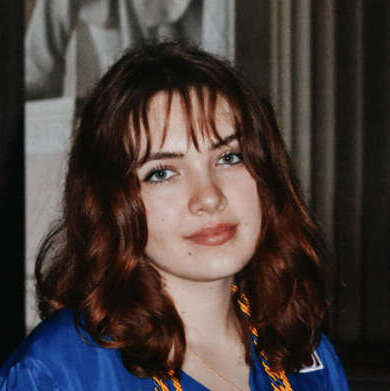